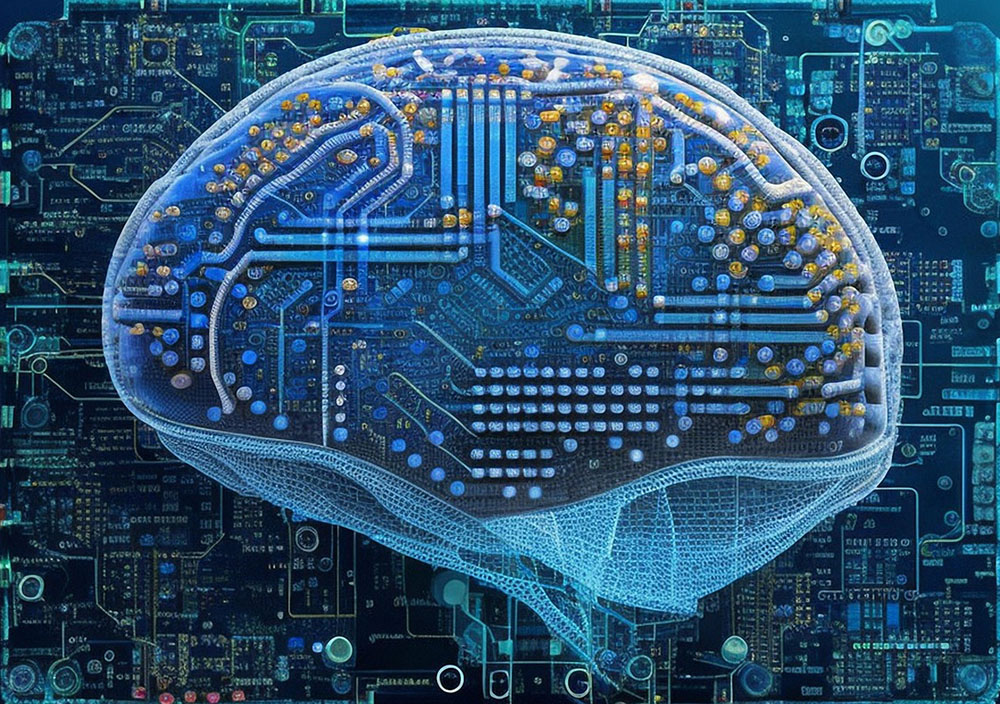
“Torture the data, and it will confess to anything.” –Ronald Coase
It has been a while since anybody at Cornerstone has seen a strategic or IT plan that has NOT said, “We will become more data-driven.” Product design/pricing, investments, marketing strategies, and almost everything else require some sort of data element.
However, financial institutions have found that they are facing a more complex environment for their data strategy every year. Multiple outsourced providers and dozens (if not more) of systems, public data, and internal data initiatives have combined to make every bank’s data environment challenging and almost always unique.
And this investment is not cheap. Recent studies by Brevo and McKinsey estimated that data costs make up roughly 5% of all non-interest expenses. This is probably a conservative number because they looked only at direct systems, people, and third-party costs. Add in the time other employees might spend creating and maintaining mini-databases and spreadsheets, balancing and reconciling information, and other activities and this number could easily be higher. The McKinsey report also found that most companies do not use 40% of the reports created for a significant length of time. Ugh.
Moreover, a recent study by Cisco estimated that corporate spending on data will increase 15% annually between 2025 and 2030. Most financial institutions would probably not disagree with that estimate.
So, the bottom line on spending is that if you buy these numbers, future spending on data initiatives will total somewhere between $1 million and $2 million annually for every $1 billion in assets.
That is an investment that requires management from every leader at the bank. The returns on this investment can be huge and are absolutely needed.
So, how do smarter banks manage this area well and get maximum investment return? Here are some of the things we have seen:
- They treat a data strategy as a corporate initiative led from the top.
Enforcing the elements of a good data strategy can’t be a responsibility dished to the CIO. While bank leadership does not need to be able to write the report or create the graph, they do need to understand and support the elements of good data design.
- They design from desired outcomes.
Nobody has the time and money to build a data warehouse and then decide what they can do with it. Smarter banks start by asking, “What are the key ‘table clearing’ reports or insights our management team needs to significantly improve performance and customer delivery?” And they keep them in mind when designing. This is not to say that IT groups don’t need to proactively design data repositories. They do, but always with the business outcome in mind.
- Repositories are kept to a minimum and synched.
My colleague Ron Shevlin has several times opined on what the right eulogy service for the “big data” craze should be. No financial institution will ever have a single, grand database. There are just too many vendors and systems in the FI ecosystem to allow that. That said, smarter banks do avoid letting everybody go wild and build a local data mart that can be repetitive and contain conflicting information. They are good at managing to the right numbers and syncing common data across them (a good AI initiative). And, they have stopped letting local Excel spreadsheets be the data repository/source of truth. I can already anticipate the emails about this.
- They get nomenclature and syntax right.
This is a way-down-in-the-weeds best practice that can’t be ignored. Smart banks create standards to classify data types, name and format data fields, and they enforce common names across different databases. Then they stick to them. This “measure twice, cut once” design for data classification pays huge dividends down the road.
- Maintaining strong data integrity is a company habit.
This means every employee. Nothing creates a lack of confidence in information faster than missing, obsolete, or just plain wrong data. And all the AI and integration in the world can’t overcome sloppy, uncorrected data input. Smart banks recognize the value in good, consistent data that holds up over time. Everybody is held accountable for this.
At the end of the day, good data management is a marathon only won by good tools, good design, good discipline, and clearly realized business outcomes. High-performing banks get this and are already seeing the payoff.